Predictive Design
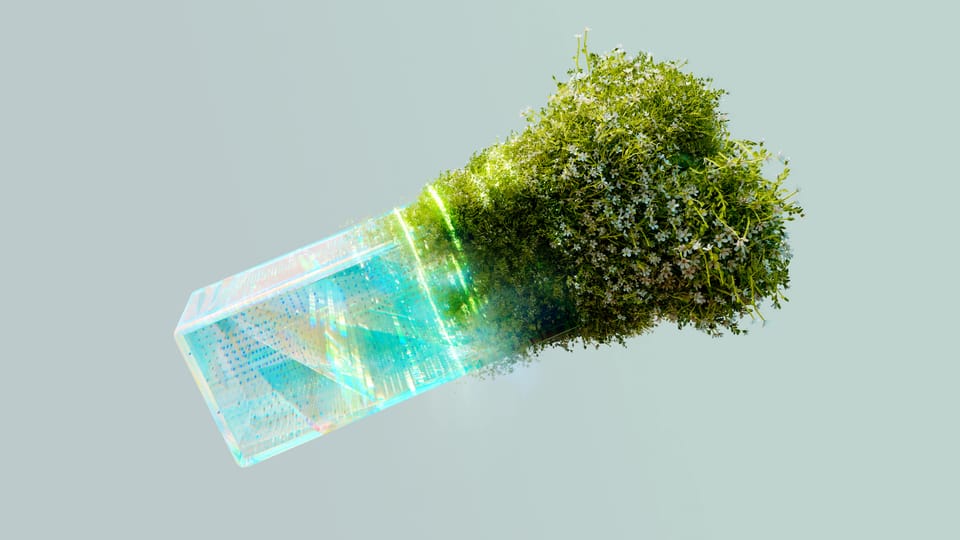
Predictive design is a human-centered approach to UX that leverages AI and data-driven insights to anticipate user needs before they take action. Instead of waiting for users to make a request, whether it’s searching for a product, adjusting settings, or navigating an app, predictive design proactively delivers relevant content, actions, and recommendations at the right time.
How Predictive Design Works
At its core, predictive design is powered by machine learning, behavioral analytics, and real-time data processing. AI models analyze patterns in user behavior, learning from past interactions to forecast future intent. This allows interfaces to dynamically adjust, creating a smoother, more intuitive user experience.
- Data collection: AI gathers data on user actions, preferences, and interactions.
- Pattern recognition: Machine learning identifies recurring behaviors and trends.
- Real-Time adaptation: The interface evolves, surfacing the most relevant options before the user searches for them.
For example, if you routinely check the weather in the morning, a predictive design system might display the forecast as soon as you unlock your phone, eliminating the need to search for it. If you frequently order coffee at a certain time, a café app might pre-fill your favorite order and prompt you with a one-tap checkout.
Examples of Predictive Design you may know:
- Smart recommendations: Netflix, Spotify, and YouTube analyze viewing and listening habits to recommend content users are likely to enjoy often before they even realize they want it.
- Predictive text & auto-suggestions: From Gmail’s Smart Compose to Google’s search predictions, AI anticipates what users are about to type and offers suggestions to reduce effort and increase efficiency.
- Adaptive interfaces: Fitness apps like Strava automatically surface relevant features like as tracking a run based on context like location, time of day, or past activity patterns.
- Proactive problem-solving: Airlines use predictive design to flag potential issues, such as notifying travelers about delays before they reach the airport or suggesting rescheduling options before a flight is canceled.
- AI-powered personal assistants: Virtual assistants like Siri, Alexa, and Google Assistant integrate predictive design to offer timely reminders, suggest responses, and anticipate user commands based on patterns.
How Predictive Design Differs from Traditional UX
Traditional UX |
Predictive Design |
---|---|
Users manually search for information |
AI anticipates needs and presents information proactively |
Interfaces remain static |
Interfaces evolve dynamically based on context |
Users follow predefined workflows |
AI optimizes workflows based on behavior patterns |
Personalization is manual (e.g., settings adjustments) |
AI automates personalization in real-time |
Instead of designing for “What does the user need right now?”, predictive design focuses on “What will the user need next?” and surfaces that information seamlessly, without friction.
Why Predictive Design Matters
As digital experiences become more complex, predictive design reduces cognitive load by streamlining interactions and making interfaces more intuitive. Well-implemented predictive UX means:
✅ Fewer clicks, faster results: AI eliminates unnecessary steps.
✅ More relevant content: Users get recommendations tailored to their actual needs.
✅ Less friction: The experience feels effortless and “just works.”
However, as predictive design becomes more pervasive, it raises ethical questions about data privacy, user autonomy, and AI bias. These issues must be carefully addressed to ensure design remains empowering, not intrusive.
Challenges & ethical considerations
While predictive design has the potential to create seamless, intuitive experiences, it also introduces complex ethical dilemmas and design challenges. As AI takes on a more proactive role in shaping user interactions, questions arise around privacy, autonomy, algorithmic bias, and the balance between convenience and control. How much personalization is too much? At what point does anticipation become intrusion? And how do we ensure that AI-driven design remains fair, transparent, and inclusive? Addressing these challenges is crucial to building predictive experiences that enhance usability without compromising user trust.
🔻 The Risk of Over-Personalization: At what point does predictive design become intrusive rather than helpful? Users may feel uncomfortable when AI knows too much—like an app predicting a pregnancy before someone shares the news.
🔻 Bias in AI models: AI is only as good as the data it learns from. If predictive algorithms are trained on biased datasets, they can reinforce exclusionary design patterns, making experiences less inclusive.
🔻 Loss of user control: Too much automation can strip users of their ability to explore freely. If predictive algorithms only serve content based on past behavior, users may end up in echo chambers, limiting discovery and creativity.
🔻 Privacy & trust: AI-driven personalization requires data. But how much should users be willing to share? Companies must balance convenience with transparency, ensuring users understand how their data is being used and offering opt-in controls.
Where do we go from here?
Predictive design isn’t about handing over creativity to AI or allowing algorithms to dictate user experiences blindly. The goal is to create experiences that feel effortless, personalized, and human, where technology anticipates needs without feeling invasive or manipulative.
To achieve this, intentionality must be at the core of predictive design. Designers and product teams must ask whether a prediction genuinely improves the user’s experience or if it removes too much control. They need to consider whether convenience is prioritized at the cost of transparency and autonomy. One of the biggest challenges in predictive design is striking the right balance between automation and user agency. While AI can streamline workflows, over-automation can make users feel powerless, as if they are being guided down a path they never chose. This is why ethical design principles, such as opt-in personalization, clear feedback loops, and the ability to override AI-driven decisions, are essential.
As AI becomes more deeply integrated into design, UX and product teams must take an active role in shaping its direction. Predictive design should be built around human psychology, not just data patterns. Users should understand why AI is making predictions and be given the ability to adjust or opt out. Systems must be trained on diverse datasets to avoid reinforcing harmful biases, ensuring that predictive experiences are fair and inclusive. AI should also improve over time based on user feedback, allowing for continuous refinement instead of static predictions. Above all, predictive design should empower users rather than control them—acting as a co-pilot that assists rather than dictates.
The next evolution of UX is not just about designing interfaces but about designing intelligence. Predictive design will shape everything from personalized healthcare recommendations to AI-driven creative tools that help users ideate faster. The challenge is to ensure that as AI-powered design becomes more intuitive, it also remains ethical, transparent, and user-centered.
Great UX isn’t just about making things look good or function well, now it’s also about making technology feel like an extension of human thinking.